Inferential based statistical indicators for the assessment of solar resource data
DOI:
https://doi.org/10.17159/2413-3051/2019/v30i1a5430Keywords:
Solar resource assessment, statistical comparison techniques, multivariate profile analysis, interval estimate plotsAbstract
The drive to reduce fossil fuel dependency led to a surge in interest in renewable energy as a replacement fuel source, which provided research opportunities for vastly different domains. Statistical modelling was used extensively to assist in research. This study applied two statistical techniques that can be used in conjunction or independently to existing methods to validate solar resource data simulated from models. The case study, using a database from a Southern African Universities Radiometric Network, provided illustrative benefits to the methods proposed, while comparing them with some of the validation methods currently used. It was demonstrated that profile analysis plots are easy to interpret, as deviations between modelled and measured data over time are clearly observed, while traditional validation scatter plots are unable to distinguish these deviations.
Downloads
References
Amillo, A.G., Ntsangwane, L., Huld, T. and Trentmann, J. 2018. Comparison of satellite-retrieved high-resolution solar radiation datasets for South Afri-ca. Journal of Energy in Southern Africa 29(2): 63-76.
https://doi.org/10.17159/2413-3051/2018/v29i2a3376
An, N., Hemmati, S. and Cui, Y.J. 2017. Assessment of the methods for determining net radiation at different time-scales of meteorological variables. Journal of Rock Mechanics and Geotechnical Engineering 9(2): 239-246.
https://doi.org/10.1016/j.jrmge.2016.10.004
Badescu, V. 2013. Assessing the performance of solar radiation computing models and model selection procedures. Journal of Atmospheric and Solar-Terrestrial Physics 105: 119-134.
https://doi.org/10.1016/j.jastp.2013.09.004
Badescu, V., Gueymard, C.A., Cheval, S., Oprea, C., Baciu, M., Dumitrescu, A., Iacobescu, F., Milos, I. and Rada, C. 2013. Accuracy analysis for fifty-four clear-sky solar radiation models using routine hourly global irradiance measurements in Roma-nia. Renewable Energy 55: 85-103.
https://doi.org/10.1016/j.renene.2012.11.037
Brooks, M.J., Du Clou, S., Van Niekerk, W.L., Gauché, P., Leonard, C., Mouzouris, M.J., Meyer, R., Van der Westhuizen, N., Van Dyk, E.E. and Vorster, F.J. 2015. SAURAN: A new resource for solar radio-metric data in Southern Africa. Journal of Energy in Southern Africa 26(1): 2-10.
Bulut, O. and Desjardins, C. 2013. Profile analysis of multivariate data in R.R package version 0.2-1. http://CRAN.R-project.org/package=profileR.
Clohessy, C. 2017. Statistical viability assessment of a photovoltaic presence of data uncertainty. Un-published PhD Thesis, Nelson Mandela Metropoli-tan University, Port Elizabeth.
David, M., Guerin de Montgareuil, A., Merten, J., Mayer, D., Proisy, B. and Olivier G. 2007. Solar resource as-sessment for PV applications. Beijing (China): Solar World) Congress; September 2007.
Davies, J.A., McKay, D.C., Luciani, G. and Abdel-Wahib, M. 1988. Validation of models estimating solar ra-diation on horizontal surfaces. Task IX final report to the solar heating and cooling programme of the In-ternational Energy Agency. Atmospheric Environ-ment Service, Downsview, Ont., Canada, IEA, Paris.
Espinar, B., Ramírez, L., Drews, A., Beyer, H.G., Zar-zalejo, L.F., Polo, J. and Martín, L. 2009. Analysis of different comparison parameters applied to solar ra-diation data from satellite and German radiometric stations. Solar Energy 83(1): 118-125.
https://doi.org/10.1016/j.solener.2008.07.009
Gueymard, C.A. 2014. A review of validation method-ologies and statistical performance indicators for modeled solar radiation data: Towards a better bankability of solar projects. Renewable and Sus-tainable Energy Reviews 39: 1024-1034.
https://doi.org/10.1016/j.rser.2014.07.117
Gueymard, C.A., Gustafson, W.T., Bender, G., Etringer, A. and Storck, P. 2012. Evaluation of procedures to improve solar resource assessments: optimum use of short-term data from a local weather station to correct bias in long-term satellite derived solar radiation time series. In World Renewable Energy Forum Confer-ence Proceedings, Denver: 13-17.
Gueymard, C.A. and Myers, D.R. 2009. Evaluation of conventional and high-performance routine solar radiation measurements for improved solar resource, climatological trends, and radiative modeling. Solar Energy 83(2): 171-185.
https://doi.org/10.1016/j.solener.2008.07.015
Iqbal, M. 2012. An introduction to solar radiation. Else-vier.
Jang, D.H. 2018. Discussion of “Graphics to facilitate informative discussion and team decision‐making”. Applied Stochastic Models in Business and Industry 34(6): 981-984.
https://doi.org/10.1002/asmb.2347
Kleissl, J. 2013. Solar energy forecasting and resource assessment. Academic Press, New York.
Kohler, M. 2014. Differential electricity pricing and ener-gy efficiency in South Africa. Energy 64: 524-532.
https://doi.org/10.1016/j.energy.2013.11.047
Kudish, A.I., Lyubansky, V., Evseev, E.G. and Ianetz, A. 2005. Inter-comparison of the solar UVB, UVA and global radiation clearness and UV indices for Beer Sheva and Neve Zohar (Dead Sea), Isra-el. Energy 30(9): 1623-1641.
https://doi.org/10.1016/j.energy.2004.04.033
Lefèvre, M., Wald, L. and Diabate, L. 2007. Using re-duced data sets ISCCP-B2 from the Meteosat satel-lites to assess surface solar irradiance. Solar Ener-gy 81(2): 240-253.
https://doi.org/10.1016/j.solener.2006.03.008
Lopez, G., Rubio, M.A., Martınez, M. and Batlles, F.J. 2001. Estimation of hourly global photosynthetically active radiation using artificial neural network mod-els. Agricultural and Forest Meteorology 107(4): 279-291.
https://doi.org/10.1016/S0168-1923(01)00217-9
Montgomery, D.C. and Runger, G.C. 1993. Gauge ca-pability and designed experiments. Part I: basic methods. Quality Engineering 6(1): 115-135.
https://doi.org/10.1080/08982119308918710
Msimanga, B. and Sebitosi, A.B. 2014. South Africa's non-policy driven options for renewable energy de-velopment. Renewable Energy 69: 420-427.
https://doi.org/10.1016/j.renene.2014.03.041
Opálková, M., Navrátil, M., Špunda, V., Blanc, P. and Wald, L. 2018. A database of 10 min average meas-urements of solar radiation and meteorological vari-ables in Ostrava, Czech Republic. Earth System Sci-ence Data 10(2): 837-846.
https://doi.org/10.5194/essd-10-837-2018
Pegels, A. 2010. Renewable energy in South Africa: Potentials, barriers and options for support. Energy Policy 38(9): 4945-4954.
https://doi.org/10.1016/j.enpol.2010.03.077
Perez, R., Ineichen, P., Moore, K., Kmiecik, M., Chain, C., George, R. and Vignola, F. 2002. A new operational model for satellite-derived irradiances: description and validation. Solar Energy 73(5): 307-317.
https://doi.org/10.1016/S0038-092X(02)00122-6
R Core Team. R: A language and environment for statis-tical computing. 2014. R Foundation for Statistical Computing, Vienna, Austria. URL http://www.R-project.org/.
Remund, J., Mueller, S., Kunz, S., Huguenin-Landl, B., Studer, C. and Schilter, C. 2014. Meteonorm Version 7.1, Handbook. Part I: Software.
Remund, J., Mueller, S., Kunz, S. and Schilter, C. 2012. Meteonorm handbook, part II: theory. Bern, Switzer-land, Meteotest.
Rencher, A.C. 2003. Methods of multivariate analy-sis (Vol. 492). John Wiley & Sons.
Sanoh, A., Kocaman, A.S., Kocal, S., Sherpa, S. and Modi, V. 2014. The economics of clean energy re-source development and grid interconnection in Af-rica. Renewable Energy 62: 598-609.
https://doi.org/10.1016/j.renene.2013.08.017
Sung, T., Yoon, S.Y. and Kim, K.C. 2015. A mathemati-cal model of hourly solar radiation in varying weath-er conditions for a dynamic simulation of the solar organic rankine cycle. Energies 8(7): 7058-7069.
https://doi.org/10.3390/en8077058
Willmott, C.J. and Matsuura, K. 2005. Advantages of the mean absolute error (MAE) over the root mean square error (RMSE) in assessing average model performance. Climate research 30(1): 79-82.
https://doi.org/10.3354/cr030079
Willmott, C.J., Matsuura, K. and Robeson, S.M. 2009. Ambiguities inherent in sums-of-squares-based error statistics. Atmospheric Environment 43(3): 749-752.
Woodall, W.H. and Montgomery, D.C. 2014. Some cur-rent directions in the theory and application of statis-tical process monitoring. Journal of Quality Tech-nology 46(1): 78-94.
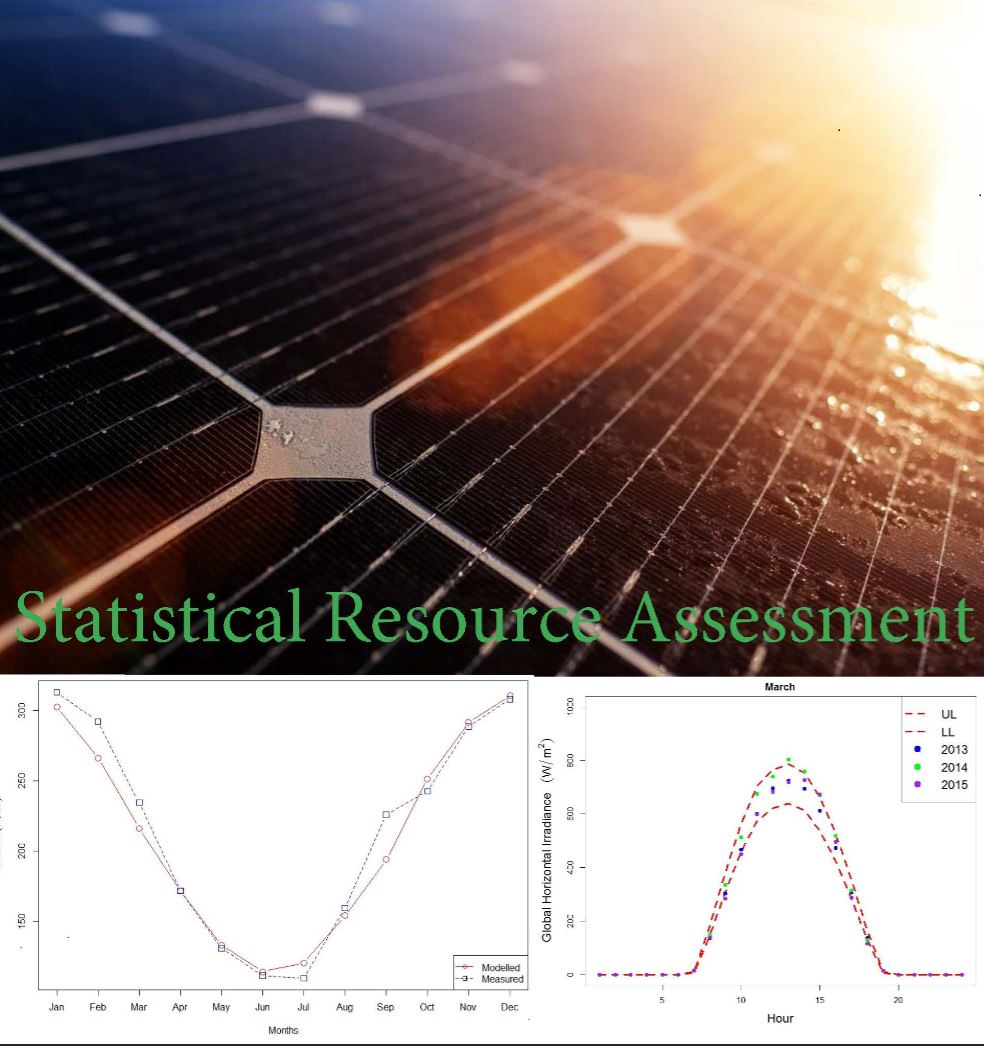
Downloads
Published
Issue
Section
License
Copyright (c) 2019 Chantelle May Clohessy, Gary Sharp, Johan Hugo, E. van Dyk

This work is licensed under a Creative Commons Attribution-ShareAlike 4.0 International License.